Today we have part two of our our series on Catastrophe Models. You can read part one here.
“You stop sending me information, and start getting me some.” Gordon Gekko
In this, the second part of our series on CAT models, we will go into the guts of a catastrophe (CAT) model and explain how they are constructed, assumptions embedded in them, and the financial consequences of these assumptions.
The foundation of actuarial premium setting generally sits on two pillars:
- How often can we expect losses to occur (the frequency of loss), and
- How large will those losses be (the severity of loss)
For much of the history of property and casualty insurance, frequency and severity were statistically inferred from claims history. The problem arises that these pillars of insurance collapse when we try to quantify losses that arise from large and significant events. This happens because large and significant events are rare by nature. Because they happen infrequently, and no two events are identical, it is extremely difficult to quantify what the actual risk is. This, not coincidentally, is the first problem a CAT model attempts to solve.
All CAT models, regardless of origin, have event catalogs that provide the data which tells us what the frequency and severity likelihood is expected to be for a given peril. These catalogs are the science behind the models. They are constructed by teams of scientists and mathematicians who, upon examining the historical events that have actually occurred, extrapolate what could occur. This is a big point, the historical data is only used as a starting point and is, by itself, not sufficient in constructing a model.
Take for instance a model for US hurricanes. The historical data from the National Hurricane Center contains data for about 150 years’ worth of storms. A wealth of information is there. But unfortunately, 150 years is not a large enough sample size for a peril such as US Hurricane to provide ample confidence for managing the risks of an (re)insurance company. This data set shows that the state of Georgia seems to get a lot less activity than Florida to the south and the Carolinas to the north. Yet, meteorologists would just explain that, scientifically, as just plain random luck more than anything else.
And that is precisely the objective of the event catalog. Its purpose is to provide a large enough sample of events that the element of luck or randomness diminishes while also maintaining the integrity of the historical data. In other words, the data we need to make critical decisions must plug up any holes that exist and still make sense based on the history we have experienced. If we are working with event catalogs that have more events making landfall in Georgia over Florida, then empirically we know that the catalog is not useful because it is not accurately representing what history tells us and what we have seen firsthand, which is Florida is much more a magnet for hurricane activity.
Another critical element is that the event catalog must tell modelers what the severity of each event is. Again, the historical catalog provides the initial guidance, and the scientists and mathematicians use their expertise to fill in the gaps. This is the portion of the event catalog that tells us if an event is a category 5 or category 1 hurricane, a magnitude 8 or magnitude 5 earthquake, an EF5 or EF1 tornado or even a 5 ton truck bomb or a 10 ton truck bomb.
Before we move on to the second component of a CAT model, there is one final element that is handled in stage 1. It’s one thing to know both the location and the strength of an event, but how does that event affect a property? What if the property is very far away from the center of the event? Very close?
This primary module of a CAT model also contains propagation or attenuation functions, which is just a fancy way of saying, mathematical equations that take source energy (whether from wind, ground shaking or some other source) and calculates what remaining energy exists by the time it reaches a property. These functions make sure that the further you are away from the eye of the storm or the epicenter of an earthquake the less intensity you should expect.
The description of the event catalog and the attenuation functions is generally known in the industry as the Hazard Module of a CAT model.
Given that CAT models estimate the level of intensity at each location, an engineering or vulnerability module is introduced that then estimates what the damage level might be based on that intensity. Engineers who build these vulnerability modules use prior claims data and whatever other scientific data they can find including studies using shake tables, wind-tunnels and additional computerized simulations.
An interesting by-product of a vulnerability module is that in the real world crazy things can and do happen. Here is an example. In the following photo, two identical buildings in Taiwan, experiencing the same earthquake had two polar loss results. One building was a total loss while the other had nearly no loss. How do we explain this? How can we make sense of this to assist us in predicting what might occur if another earthquake were to strike this area (or any other).
This issue is termed secondary uncertainty, and it is the general inability to pin down a damage estimate because of unknown factors that creep into a model. Perhaps the soil for one building is significantly different than the building across the street; perhaps each building was built by two different builders one of whom decided to cut a few corners. These are a couple of the many unknowns that engineers must deal with when examining the historical data. This is also the reason why a model cannot be used to accurately estimate loss for a single event. There are just too many unknowns that get into the process and the best we can do, at the end of the day, is to categorize the degree of confidence we have when each of these types of events occur. With a large enough portfolio and event set, we can only hope some of the uncertainty cancels itself out. (Article 3 in this series discusses the uncertainty and what that means for decision making).
It is at this stage that an insurer’s actual property portfolio interfaces with the model. In order to have a high degree of confidence, an insurer should know at least the following characteristics about a property:
- Construction – This attribute of a property can significantly affected modeled losses. Wood frame homes behave differently than masonry. Steel framed structures are much stronger than light metal.
- Occupancy – What is the structure used for? Office towers are generally highly engineered structures as compared to a simple residential home. Industrial facilities are quite complicated and damage to critical components could shut down a site for an extended period of time, exacerbating a loss.
- Age – Older structures are vulnerable to construction codes that were non-existent or weaker than those that exist today.
- Height – As buildings get taller, they generally become more vulnerable. As you can see in the shake table video, as a building begins to sway, the upper floors amplify the swaying and are the first components to potentially fail.
- Geo-resolution – Do we know precisely where the location is? If not, the model will be making some pretty wild guesses about where it might be, and the conditions might be drastically different than the actual location.
These are just five of hundreds of different property characteristics that can be plugged into a CAT model. The big idea here is that data means everything. Even with good quality data, I have already escribed how a lot of uncertainty gets into modeled estimates, so insurers that are not capturing their property exposures correctly are at a competitive disadvantage compared to their peers.
The final component of a CAT model is the Financial Module:
This is the portion of the CAT model that applies the financial terms to the damage estimates of the Damage module. Even at this stage many assumptions must be made that can have consequential effects on final loss estimates. Most of these assumptions are beyond the scope of this article and more applicable for our actuarial friends. This is the one module of a CAT model where the assumptions can have a consequential effect on loss estimates but requires a high degree in mathematics to fully understand those assumptions and to be able to run sensitivities off of them. Every modeling team should work with the actuarial and the capital modeling department to make sure that the financial assumptions are acknowledged and fit the criteria for the firm.
The general point that I hope I have amply stressed in this article, is that there are a lot of assumptions required to build and run a CAT model. Models work best when we are using them as a tool to generate information that we can then make decisions off of. When it comes to CAT models, you can NOT take the loss estimates to the bank. Everything must be questioned and critiqued.
And yet, even with all the warts, CAT models have proven themselves to be a worthy tool. Those insurers who were the early adopters got a significant competitive advantage. Now that the models are ubiquitous in the industry, that competitive advantage has disappeared as even the smallest of firms has access to some amazing technology. There is no going back.
In the next article in the series, I will explore the output of CAT models and hint at some areas where early adopters can find a new competitive advantage. (Go to article 3 in this series: https://insnerds.com/using-catastrophe-models/)
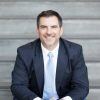
About Nicholas Lamparelli
Nick Lamparelli is a 20+ year veteran of the insurance wars. He has a unique vantage point on the insurance industry. From selling home & auto insurance, helping companies with commercial insurance, to being an underwriter with an excess & surplus lines wholesaler to catastrophe modeling Nick has wide experience in the industry. Over past 10 years, Nick has been focused on the insurance analytics of natural catastrophes and big data. Nick serves as our Chief Evangelist.
Nick Lamparelli is a 20+ year veteran of the insurance wars. He has a unique vantage point on the insurance industry. From selling home & auto insurance, helping companies with commercial insurance, to being an underwriter with an excess & surplus lines wholesaler to catastrophe modeling Nick has wide experience in the industry. Over past 10 years, Nick has been focused on the insurance analytics of natural catastrophes and big data. Nick serves as our Chief Evangelist.