This article originally appeared on InsNerds.com
“The information you have is not the information you want. The information you want is not the information you need. The information you need is not the information you can obtain. The information you can obtain costs more than you want to pay”
― Peter L. Bernstein, Against the Gods: The Remarkable Story of Risk
In the world of property insurance, little of significance gets done without the information generated with catastrophe (CAT) models. From reinsurance treaties to CAT bonds, underwriting, pricing and even claims, CAT models now touch nearly every facet or property insurance. But at the end of the day, is the information that comes out of these CAT models what property insurers want or need? How useful is this information and why is the industry so beholden to it?
I will spend three separate articles describing the tradeoffs of CAT models; what information it provides, how it generates this information, why we need it and most importantly, how to use it. In this, the first of three, I argue that CAT models, at a minimum, give us the information that we once wanted.
Why Do We Need CAT Models?
Insurance products are generally priced assuming that the overall number of claims and the amount of losses stemming from those claims can accurately be predicted (otherwise, why bother being in business). This is due to the concept of the law of large numbers, which means that as the absolute number of individual policies issued increases, the more confidence we can have in being able to estimate losses for the entire group or portfolio, which means more confidence in pricing the risk and managing the risks correctly.
So for example, estimating the likelihood of auto damage to an entire portfolio of 100,000 cars is enormously easier than for a single car. Over time, losses from a large portfolio of exposures begin to develop into patterns or distributions. This is where our handy actuaries come in. They crunch volumes of historical exposures and losses to develop those distributions so that the patterns of losses within them permit us to predict how losses will occur in the future to a similar set of exposures. Once we are confident of what the future losses will reasonably look like, we can set a rate or price that will adequately pay for those future losses with hopefully enough left over to adequately compensate the insurer for risks taken. This works for auto insurance but it is also how fire, life and most other insurers operate.
Life would be good if this was all insurance was all about, but unfortunately two recurring problems have revealed themselves, which have continually brought many insurance companies to their knees:
- Concurrent claims – events that occur that unexpectedly affect a large number of policyholders simultaneously, and
- Lack of historical data – the inability to use or find relevant prior loss data to guide confidence in estimating future losses.
When these two problems occur, standard actuarial techniques, as described above, are not adequate. Unfortunately, devastating natural phenomenon such as earthquakes, hurricanes, floods and other severe storms have these exact criteria.
CAT Models Generate Information
What do all of these hypothetical events have in common?:
– A magnitude 9 earthquake and tsunami off the coast of Seattle, WA
– A hurricane making a landfall on the Georgia coast
– A category 4 hurricane making a landfall in New York City
What all of these events have in common is that, for starters, they would be consequential in property damage and loss of life. What is even more striking is that we have no recorded history of any of these events ever occurring…but they all have occurred or are expected to occur.
So, if you are a property insurer, trying to develop a reasonable premium for wind exposure for a property in Savannah, GA or New York City, wouldn’t you want to know the likelihood of a hurricane and the strength of that hurricane striking your exposures? Of course!
Unlike fire risk, the historical record, by itself is inadequate in providing the necessary guidance for a property insurer to estimate what future losses will look like. Not to mention how a portfolio of properties might behave when a hurricane, earthquake or tornado strikes multiple properties simultaneously.
Enter the CAT model. The way I like to describe CAT models is that it is a way to build the claims data, where none exist. I will spend much of article 2 describing the guts of a CAT model, but for simplicity it is good enough to think about them as a set of computerized instructions, built by scientists, engineers, mathematicians and actuaries that simulate thousands to millions of years of potential catastrophic events.
I bet you already knew that earthquake risk is greater in Los Angeles than in Omaha, Nebraska but did you know that earthquake risk in New York City ispotentially greater than that in Los Angeles? It’s true. The models point out that while New York City has far fewer and less severe earthquakes than Los Angeles, the building codes are also far weaker and those brick and masonry homes will become very brittle when a large earthquake does finally strike New York City.
Did you know that the seismic risk in Charleston, SC and Little Rock AK is likely higher than in San Diego or Sacramento, CA? Or than inland states such as Tennessee or West Virginia are susceptible to hurricane losses?
If you are a property insurer writing large lines with high attachment points in Miami, FL, it was nearly impossible to estimate what your hurricane exposure was before the advent of CAT models. Now, the models can estimate the frequency and severity of your exposure and estimate when and how often these potential hurricane losses might make it into your layer.
CAT models provide guidance for a whole range of questions surrounding frequency and severity of losses due to wind, rain, hail, flooding, earthquakes, and even man-made events such as terrorism. In fact, because of computerized technology, CAT models will solve both of the problems I mentioned above; it will provide the data that is missing from the historical losses AND provide portfolio statistics for all of locations as events strike swaths of your exposures. If you are an underwriter, writing business up and down the east coast, CAT models will estimate how events affect all of your locations simultaneously as it simulates each of the thousands of hurricane tracks with the models catalog of events. In effect, you will know what you can expect on average, for both your portfolio and individual locations and will guide you as how probable certain loss levels are to be reached or exceeded.
Here are some questions that CAT models provide direction on:
– What are my losses likely to be on average for a policy year? How about for the entire portfolio?
– How likely is it that we will experience a $10 million, $100 million, $1billion loss? Where are these losses likely to come from? How does that affect our risk appetite?
– How does risk associated with property A compare with property B? Region A versus region B?
– What are the property lines or regions where the market is mispricing or incorrectly underwriting the exposures? Can we develop a competitive advantage?
– How would our losses change if we add coverage for flood or earthquake risks? Will the market support the price we will need to charge for these new exposures? How would that affect our reinsurance costs and agency ratings?
These are just a few of the multitude of questions I have had to answer using data derived from CAT models.
Put simply, CAT models have positioned the insurance industry in a much better place by making those that write property business better informed. Property insurers that minimize or neglect the use of CAT models are essentially bringing a knife to a gunfight.
Brokers and agents that are not using CAT models are doing a disservice to their customers by not arming them with the information they need to make the best risk transfer decisions? How can a broker possibly advise on retention and limits if they have no idea of what the loss profile will look like? Those decisions are arbitrary and foolish in the face of all of the uncertainty that the models are providing guidance on. For those brokers, they are vulnerable to losing their clients to brokers that get it; knowledge is power!
Unfortunately, CAT models are not the end all and be all of property insurance. They are providing the information we once desperately needed, but now the paradigm has changed again. In an era where most property insurers are using the same CAT models, how do you differentiate yourselves?
We now need different information. How can we make these models better? How can we manage our businesses more effectively? How can we build a risk appetite that satisfies our stakeholders and provides us with a competitive advantage? How can we create long-lasting value?
In the next set of articles, I will expand on CAT models, but also hope to answer these questions posed. (Go to article 2 in this series: https://insnerds.com/understanding-catastrophe-models/)
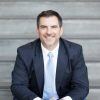
About Nicholas Lamparelli
Nick Lamparelli is a 20+ year veteran of the insurance wars. He has a unique vantage point on the insurance industry. From selling home & auto insurance, helping companies with commercial insurance, to being an underwriter with an excess & surplus lines wholesaler to catastrophe modeling Nick has wide experience in the industry. Over past 10 years, Nick has been focused on the insurance analytics of natural catastrophes and big data. Nick serves as our Chief Evangelist.
Nick Lamparelli is a 20+ year veteran of the insurance wars. He has a unique vantage point on the insurance industry. From selling home & auto insurance, helping companies with commercial insurance, to being an underwriter with an excess & surplus lines wholesaler to catastrophe modeling Nick has wide experience in the industry. Over past 10 years, Nick has been focused on the insurance analytics of natural catastrophes and big data. Nick serves as our Chief Evangelist.