(Para Español – Aqui)
In October 2015, a powerful Saffir-Simpson Category 5 tropical cyclone made landfall on the Pacific coast of Mexico, about 200 km south of Puerto Vallarta. That was hurricane Patricia, which caused an estimated USD 350 Million in uninsured damages, mainly to infrastructure, housing, and farms. Insured losses were even less, due to the storm making landfall near Cuixmala, an area with dispersed population and sparse economic activity.
Fast forward eight years, and the situation is a different one, with losses estimated to run 10 to 30 times higher than its 2015 predecessor. Last week, Hurricane Otis outpaced Hurricane Patricia with its rapid intensification, increasing windspeed by 80 mph in a period of 12-hours, according to Philip Klotzbach, a research scientist in the Department of Atmospheric Science at Colorado State University specializing in Atlantic basin seasonal hurricane forecasts. Otis surprised residents who were subjected to intense winds that tore apart windows and even the outside wall envelopes of many structures. Many buildings in Acapulco, it turns out, were designed to withstand earthquake shaking, and for this reason used lighter materials in their outside walls. In an earthquake, this reduces the weight of the building, decreases stress caused by shaking, and minimizes subsequent damage. But in a major hurricane like Otis, it was a recipe for disaster where thin walls, in some cases comprised of light sheetrock, were no match for hurricane force winds.
This rapid intensification was not anticipated by weather forecast predictions used by meteorologists to issue warnings to residents. This was confusingly referred to as a “monumental miss” in an online trade journal editorial. Except it wasn’t. Not from an insurance perspective. The weather forecasts that “missed” a component of Otis’s development are not typically used by the insurance industry in any way to model loss.
Nevertheless, Hurricane Otis was well anticipated and represented by catastrophe models used by the insurance industry. The fact that some tropical cyclones will rapidly intensify their wind speeds as the central pressure drops is immaterial to whether or not a catastrophe model correctly measures the risk of loss to property in any given year. For the latter purpose, it is much more material to focus on the frequency and severity validations related to major category hurricanes in a given geographic region, such as the Pacific coast of Mexico.
For insurance executives not close to the catastrophe loss modeling practice, the following is a primer on what can be known in the early days, when it is very early in the recovery and relatively little is known concretely about the claims that will be filed following the event.
- A reputable catastrophe model, preferably one vetted and used by large reinsurance firms, will be a credible tool for modeling insured loss both before and after the event. Two firms, Verisk (formerly known as AIR Worldwide) and RMS Moody’s are considered to offer the most robust resources and typically issue additional data and tools following major events.
- So called, Similar Stochastic Events (also referred to in industry jargon as SSEs), are the first representations of a given hurricane, such as Hurricane Otis, that are available for modeling insured losses.
A catastrophe model works by simulating next year’s hurricane season ten, fifty, or one hundred thousand times. In each of those simulation years—just like any random actual year of cyclone activity—you may have more storms or fewer storms, different tracks those storms may travel, and different territories affected. It’s out of that large series of simulations that loss scenarios are generated, and statistics such as an Average Annual Loss for a given single-risk or portfolio of properties may be developed.
The potential downside of using SSEs is that they represent merely one sampling of an almost infinite collection of plausible storms for a given geography. While a catalog’s completeness should correspond closely to the full range of physically possible/probable event intensities for a given coastal segment, there are many detailed parameters that may actually be different from those observed in a particular storm like Otis. For major hurricane events, there is a solution that helps companies reduce uncertainty and more accurately model the loss.
- Cat-modeling companies will produce bespoke, tailored event representations that allow for re/insurers and brokers to model the loss of the recently observed event. Verisk, for example, will produce an ALERT event set which comprises five representations of the event which are more tightly coupled to both the physical parameters (representations of wind-field size, wind speeds, levels of precipitation-induced flooding) and tuned to the physical levels of damage that early reports suggest. As following an event there are many uncertainties in terms of the observed wind speeds and meteorological parameters, the range of five scenarios captures a reasonable range of potential losses either for a single policy or an entire insured portfolio of risks. A note of caution: it is tempting for executives to take hold of the scenario modeling the highest level of loss and for that estimate to be rigorously interrogated. However, until well after the event, it will not be known which of the 5 bespoke events will best represent the loss for any given portfolio. It’s best to carefully discern the nature of the portfolio in question to more accurately evaluate the simulated loss.
- Gold-IN, Gold-OUT. (likewise, garbage-IN and garbage-OUT)
This lesson holds for the inputs used in any catastrophe loss analysis. The primary input is the Exposure Data which is the representation of the insured set of buildings being analyzed for loss.
For a model to accurately analyze the losses to a given set of exposures, ideally the following are accurately captured:
- Replacement Values associated to each insured structure
- Accurate information reflecting policy terms, including any limits and deductibles and reinsurance coverages
- Construction material type
- Occupancy type
- Location. This may be represented at an aggregated level, such as at a Postal Code, or City or CRESTA zone. Alternatively, for individual risks the models can accurately interpret street-level geocodes (latitude-longitude coordinates) that allow a model to more accurately predict unique event impacts which can increase or decrease expected losses. For example, one address may be more vulnerable to flooding or amplified earthquake shaking due to the topography, soil types, and proximity to water sources.
Likewise, where there is uncertainty regarding the replacement values, location, or construction mix of the exposure, the modeled loss estimates should be treated carefully, as those original uncertainties can influence a loss estimation to produce unintuitive results. “Go to the source” is a powerful adage underpinning an accurate interpretation of modeled loss results.
Even before the debris cleanup begins in earnest, a reliable estimate of insured losses from the catastrophic event may be developed. Keep in mind: 1. To use a reputable, robust catastrophe model 2. SSEs may provide early guidance on loss ranges 3. For major events, bespoke representations of the event will allow analysts to reduce uncertainty in the loss estimates. 4. Gold-in. Gold-out. Mind the quality of your exposure inputs as these can greatly influence and help to explain the loss analysis results.
This primer was meant to help insurance executives recognize what is typically possible to model, loss-wise, following a major catastrophic event such as Hurricane Otis. I covered broadly what tools and data are used to analyze loss and noted a few of the potential areas of uncertainty where executives will be well-advised to be careful about what data is being used to represent the exposure of any given portfolio of insured risks. The topic of uncertainty and best practices in catastrophe risk modeling is a larger one that I plan to tackle in greater depth in a subsequent series of articles.
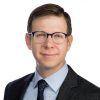
Senior Manager, Extreme Event Solutions at Verisk